1、(1)上文介绍了DDPM生成图片的原理和代码测试结果,训练时给样本图片加上gaussian noise,预测时也是预测gaussian noise;
- 这里为啥要用gaussian distribution?为啥不用其他的分布?
- 高斯分布相对比较简单,只有两个参数:均值和方差,容易控制;
- 为啥一张随机生成的gaussion noise经过很多次裁剪后能得到想要的图片?数学上的依据是什么?
- 理论上讲: 任意K个高斯分布按照特定的权重组合,能得到任意曲线,也就是拟合任意的分布
-
- 假设有K个高斯分布,这K个高斯分布称作混合模型的隐变量则复杂分布的概率分布是:
通过这种Gaussian分布拟合任意分布,这下知道为啥diffusion模型会使用上千个Gaussian noise来生成 image了吧?本质就是利用Gaussian分布的组合生成所需image!两个乘数一个是DDPM中的alpha,另一个是epsilon(预测noise的网络).一张样本图片,比如是3 channel * 28 width * 28 height = 2352个像素点。理论上讲,只要有一个像素点不同,图片就不同。再说直白一点:每个像素点的值刚开始都是随机生成的,生成的值符合Gaussian~(0,1)分布;后续迭代很多次(因为是Gaussian~(0,1)分布,每次生成的数值都较小,99.7%的数值会在(-3,3)之间。为了满足channel的数值范围(一般是0~255),需要多次迭代。比如channel的数值是240,随机生成的noise值是3,那么至少迭代80次才能满足要求),每次迭代都会用新生成的值(也符合Gaussian分布)加减初始值,直到迭代结束(这个思路和"小步快跑"的梯度下降没任何区别)。2352个像素点拼接起来就生成了预测的noise图片!
(2)怎么求seta?以VAE为例,整个网络结构如下:
Z是隐变量,经过seta网络生成X;如果这个生成的X和原来数据集一样,说明seta是正确的。那么既然x已经发生了,最合理的思路就是让X的概率最大化了,也就是max likelyhood!也就是seta网络要让X生成的概率最大,以此来得到最合适的seta网络参数!
2、实战时,肯定是要加入用户输入的prompt的!怎么严格按照用户的prompt生成image了?transformer架构最初是用来做翻译的,encoder把一种语言的输入转成embedding后,通过cross attention的机制把输入信息转移到decoder用于生成输出的token,这里也需要把输入的prompt信息传递到图片的decoder部分,是不是也能借鉴一下这个思路了?
用户输入的prompt经过矩阵的线性转换后生成了embedding,在每个resnetblock后都加上一个transformer block(down 和 up 都要加),通过这种方式把用户的prompt信息融入整个unet网络!
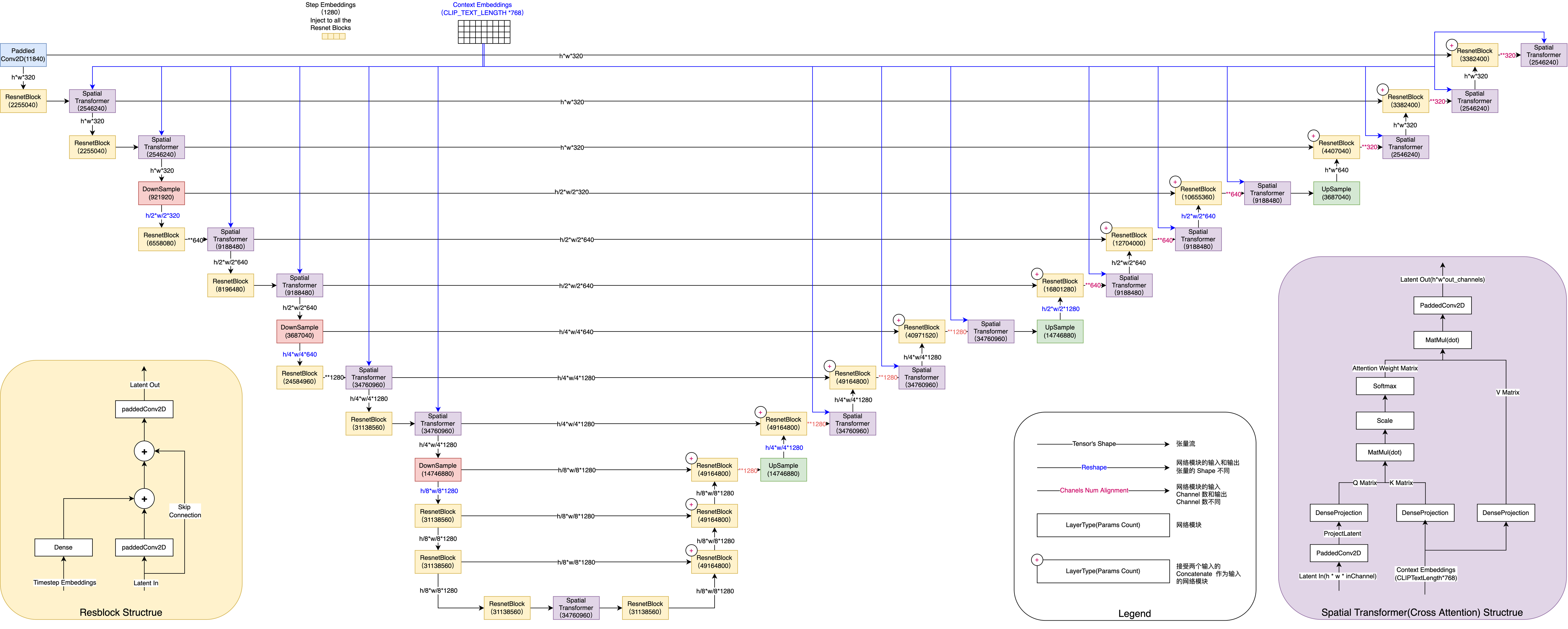
具体代码实现,参考如下:像素点是query,prompt是key和value,做cross attention!注意:为啥要用像素点做attention?生成最终的image,需要每个像素点都参与,只有每个像素点的值对了,最终的image才能正确!为了确保每个像素点都正确,需要把prompt的值融入!
import torch from torch import nn from config import * import math class CrossAttention(nn.Module):def __init__(self,channel,qsize,vsize,fsize,cls_emb_size):super().__init__()self.w_q=nn.Linear(channel,qsize)self.w_k=nn.Linear(cls_emb_size,qsize)self.w_v=nn.Linear(cls_emb_size,vsize)self.softmax=nn.Softmax(dim=-1)self.z_linear=nn.Linear(vsize,channel)self.norm1=nn.LayerNorm(channel)# feed-forward结构self.feedforward=nn.Sequential(nn.Linear(channel,fsize),nn.ReLU(),nn.Linear(fsize,channel))self.norm2=nn.LayerNorm(channel)def forward(self,x,cls_emb): # x:(batch_size,channel,width,height), cls_emb:(batch_size,cls_emb_size)x=x.permute(0,2,3,1) # x:(batch_size,width,height,channel)# 像素是QueryQ=self.w_q(x) # Q: (batch_size,width,height,qsize)Q=Q.view(Q.size(0),Q.size(1)*Q.size(2),Q.size(3)) # Q: (batch_size,width*height,qsize) 每个像素点都要参与attention的计算# prompt是Key和ValueK=self.w_k(cls_emb) # K: (batch_size,qsize)K=K.view(K.size(0),K.size(1),1) # K: (batch_size,qsize,1)V=self.w_v(cls_emb) # V: (batch_size,vsize)V=V.view(V.size(0),1,V.size(1)) # v: (batch_size,1,vsize)# attention打分矩阵Q*Kattn=torch.matmul(Q,K)/math.sqrt(Q.size(2)) # attn: (batch_size,width*height,1)attn=self.softmax(attn) # attn: (batch_size,width*height,1)# print(attn) # 就一个Key&value,所以Query对其注意力打分总是1分满分# attention输出Z=torch.matmul(attn,V) # Z: (batch_size,width*height,vsize)Z=self.z_linear(Z) # Z: (batch_size,width*height,channel)Z=Z.view(x.size(0),x.size(1),x.size(2),x.size(3)) # Z: (batch_size,width,height,channel)# 残差&layerNormZ=self.norm1(Z+x)# Z: (batch_size,width,height,channel)# FeedForwardout=self.feedforward(Z)# Z: (batch_size,width,height,channel)# 残差&layerNormout=self.norm2(out+Z)return out.permute(0,3,1,2)if __name__=='__main__':batch_size=2channel=1qsize=256cls_emb_size=32cross_atn=CrossAttention(channel=1,qsize=256,vsize=128,fsize=512,cls_emb_size=32)x=torch.randn((batch_size,channel,IMG_SIZE,IMG_SIZE))cls_emb=torch.randn((batch_size,cls_emb_size)) # cls_emb_size=32 Z=cross_atn(x,cls_emb)print(Z.size()) # Z: (2,1,48,48)
把attention机制打包到convblock中:
from torch import nn from cross_attn import CrossAttentionclass ConvBlock(nn.Module):def __init__(self,in_channel,out_channel,time_emb_size,qsize,vsize,fsize,cls_emb_size):super().__init__()self.seq1 = nn.Sequential(nn.Conv2d(in_channel,out_channel,kernel_size=3,stride=1,padding=1), # 改通道数,不改大小 nn.BatchNorm2d(out_channel),nn.ReLU(),)self.time_emb_linear=nn.Linear(time_emb_size,out_channel) # Time时刻emb转成channel宽,加到每个像素点上self.relu=nn.ReLU()self.seq2=nn.Sequential(nn.Conv2d(out_channel,out_channel,kernel_size=3,stride=1,padding=1), # 不改通道数,不改大小 nn.BatchNorm2d(out_channel),nn.ReLU(),)# 像素做Query,计算对token的attention,实现分类信息融入图像,不改变图像形状和通道数self.crossattn=CrossAttention(channel=out_channel,qsize=qsize,vsize=vsize,fsize=fsize,cls_emb_size=cls_emb_size)def forward(self,x,t_emb,cls_emb): # t_emb: (batch_size,time_emb_size)x=self.seq1(x) # 改通道数,不改大小t_emb=self.relu(self.time_emb_linear(t_emb)).view(x.size(0),x.size(1),1,1) # t_emb: (batch_size,out_channel,1,1) output=self.seq2(x+t_emb) # 不改通道数,不改大小return self.crossattn(output,cls_emb) # 图像和prompt embedding做attention
3、模型微调:市面上可能有已经训练好的模型,但模型的训练数据大概率是通用的数据,并不是某些垂直细分领域的数据,怎么才能加上自己所需垂直领域的数据了?最合适的当然是微调了!微调的方式也有很多:全量参数微调、冻结部分参数微调、lora微调。如果训练数据有限、算力也有限,那么最合适的就是lora微调了!理论上讲,任何线性变换(直白一点就是矩阵乘法啦)都可以旁挂两个m*r和r*n的小矩阵来完成lora微调!但是:这种任务的核心是根据prompt生成image(所以微调的样本肯定也有配对的prompt和image),重点就是融合prompt和image的cross attention了,所以这里直接在cross attention的矩阵乘法旁边外挂新矩阵来达到融合新样本信息的目的!
先找到需要旁挂小矩阵的层:
from unet import UNet from dataset import train_dataset from diffusion import forward_diffusion from config import * import torch from torch import nn from torch.utils.data import DataLoader from torch.utils.tensorboard import SummaryWriter import os from lora import inject_loraEPOCH=200 BATCH_SIZE=400if __name__=='__main__':# 加载模型model=torch.load('model.pt')# 向nn.Linear层注入Lorafor name,layer in model.named_modules():name_cols=name.split('.')# 找到cross attention中的线性变换,也就是矩阵乘法filter_names=['w_q','w_k','w_v']if any(n in name_cols for n in filter_names) and isinstance(layer,nn.Linear):inject_lora(model,name,layer)# lora权重的加载try:restore_lora_state=torch.load('lora.pt')model.load_state_dict(restore_lora_state,strict=False)except:pass model=model.to(DEVICE)# 冻结非Lora参数for name,param in model.named_parameters():if name.split('.')[-1] not in ['lora_a','lora_b']: # 非lora部分不计算梯度param.requires_grad=Falseelse:param.requires_grad=Truedataloader=DataLoader(train_dataset,batch_size=BATCH_SIZE,num_workers=4,persistent_workers=True,shuffle=True) # 数据加载器 optimizer=torch.optim.Adam(filter(lambda x: x.requires_grad==True,model.parameters()),lr=0.001) # 优化器只更新Lorac参数loss_fn=nn.L1Loss() # 损失函数(绝对值误差均值)print(model)writer = SummaryWriter()model.train()n_iter=0for epoch in range(EPOCH):last_loss=0for batch_x,batch_cls in dataloader:# 图像的像素范围转换到[-1,1],和高斯分布对应batch_x=batch_x.to(DEVICE)*2-1# 引导分类IDbatch_cls=batch_cls.to(DEVICE)# 为每张图片生成随机t时刻batch_t=torch.randint(0,T,(batch_x.size(0),)).to(DEVICE)# 生成t时刻的加噪图片和对应噪音batch_x_t,batch_noise_t=forward_diffusion(batch_x,batch_t)# 模型预测t时刻的噪音batch_predict_t=model(batch_x_t,batch_t,batch_cls)# 求损失loss=loss_fn(batch_predict_t,batch_noise_t)# 优化参数 optimizer.zero_grad()loss.backward()optimizer.step()last_loss=loss.item()writer.add_scalar('Loss/train', last_loss, n_iter)n_iter+=1print('epoch:{} loss={}'.format(epoch,last_loss))# 保存训练好的Lora权重lora_state={}for name,param in model.named_parameters():name_cols=name.split('.')filter_names=['lora_a','lora_b']if any(n==name_cols[-1] for n in filter_names):lora_state[name]=paramtorch.save(lora_state,'lora.pt.tmp')os.replace('lora.pt.tmp','lora.pt')
旁挂两个小矩阵的实现:
from config import * import torch from torch import nn import math # Lora实现,封装linear,替换到父module里 class LoraLayer(nn.Module):def __init__(self,raw_linear,in_features,out_features,r,alpha):super().__init__()self.r=r self.alpha=alphaself.lora_a=nn.Parameter(torch.empty((in_features,r)))self.lora_b=nn.Parameter(torch.zeros((r,out_features)))nn.init.kaiming_uniform_(self.lora_a,a=math.sqrt(5))self.raw_linear=raw_lineardef forward(self,x): # x:(batch_size,in_features)raw_output=self.raw_linear(x) lora_output=x@((self.lora_a@self.lora_b)*self.alpha/self.r) # matmul(x,matmul(lora_a,lora_b)*alpha/r)return raw_output+lora_outputdef inject_lora(model,name,layer):name_cols=name.split('.')# 逐层下探到linear归属的modulechildren=name_cols[:-1]cur_layer=model for child in children:cur_layer=getattr(cur_layer,child)#print(layer==getattr(cur_layer,name_cols[-1]))lora_layer=LoraLayer(layer,layer.in_features,layer.out_features,LORA_R,LORA_ALPHA)setattr(cur_layer,name_cols[-1],lora_layer)
总结:
1、 机器学习核心是根据输入数据得到所需的输出数据,肯定要对输入数据做各种转换,常见的做法就是matrix multi、active、attention等:
- matrix multi:旧的向量转移到新的空间
- 通过更改matrix的参数让新向量的数值适配下游任务
- 向量长度做调整适配
- active:特征多维组合后生成新特征,用于下游任务
- attention:相似度的计算,用于不同网络之间的信息传递与融合
参考:
1、https://www.bilibili.com/video/BV19H4y1G73r/?spm_id_from=333.337.search-card.all.click&vd_source=241a5bcb1c13e6828e519dd1f78f35b2
2、https://nn.labml.ai/diffusion/stable_diffusion/model/unet.html
3、https://deepsense.ai/diffusion-models-in-practice-part-1-the-tools-of-the-trade/
4、https://aitechtogether.com/python/77485.html