文章目录
- 过程
- 代码
- 参考
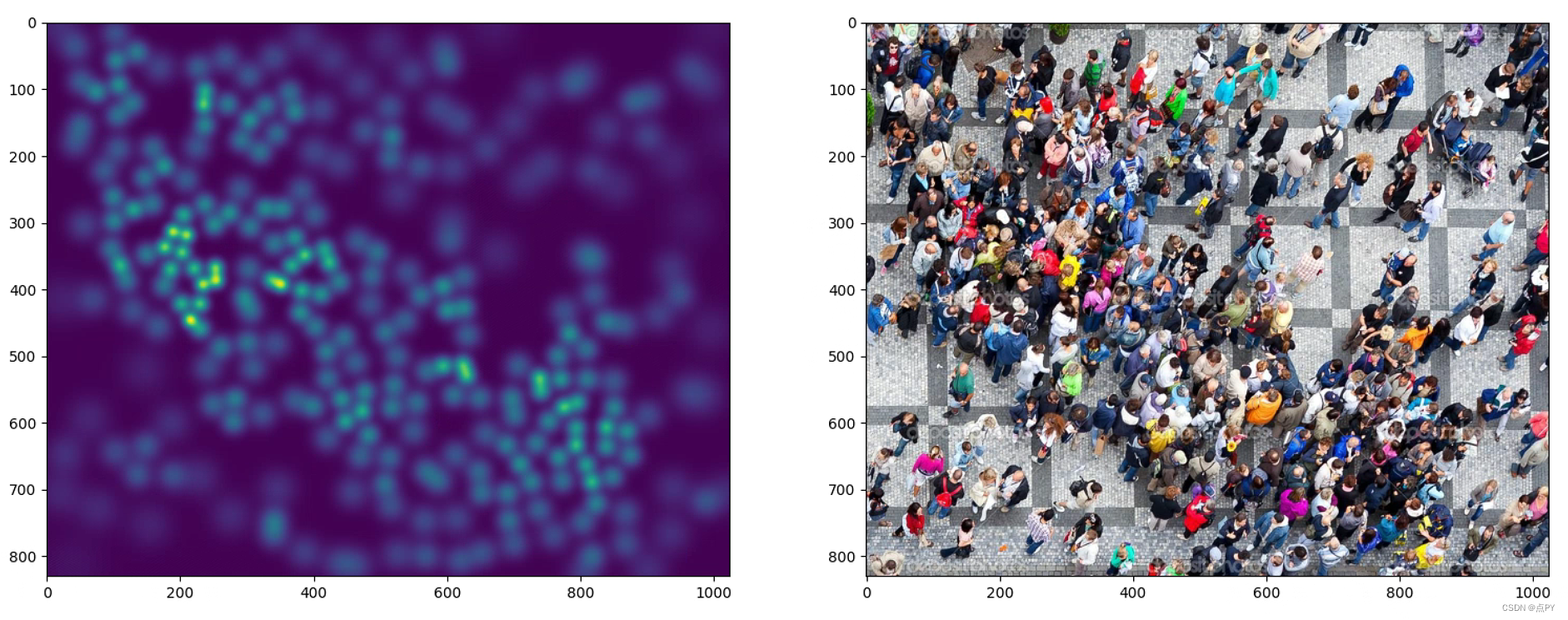
过程
首先构造一个和原始图片大小相同的矩阵,并将其全部置为0,然后将每个被标记的人头对应的位置置为1,这样就得到了一个只有0和1的矩阵,最后通过高斯核函数进行卷积得到一个连续的密度图。
代码
import h5py
import scipy.io as io
import PIL.Image as Image
import numpy as np
import os
import glob
from matplotlib import pyplot as plt
from scipy.ndimage.filters import gaussian_filter
from scipy.spatial import KDTree
import scipy
import json
from matplotlib import cm as CM
import torch#this is borrowed from https://github.com/davideverona/deep-crowd-counting_crowdnet
def gaussian_filter_density(gt):print(gt.shape)density = np.zeros(gt.shape, dtype=np.float32)gt_count = np.count_nonzero(gt)if gt_count == 0:return densitypts = np.array(list(zip(np.nonzero(gt)[1], np.nonzero(gt)[0])))leafsize = 2048# build kdtreetree = KDTree(pts.copy(), leafsize=leafsize)# query kdtreedistances, locations = tree.query(pts, k=4)print('generate density...')for i, pt in enumerate(pts):pt2d = np.zeros(gt.shape, dtype=np.float32)pt2d[pt[1],pt[0]] = 1.if gt_count > 1:sigma = (distances[i][1]+distances[i][2]+distances[i][3])*0.1else:sigma = np.average(np.array(gt.shape))/2./2. #case: 1 pointdensity += scipy.ndimage.filters.gaussian_filter(pt2d, sigma, mode='constant')print('done.')return densityif __name__ == "__main__":#set the root to the Shanghai dataset you downloadroot = r'D:\dl_dataset\object_counting\ShanghaiTech_Crowd_Counting_Dataset'#now generate the ShanghaiA's ground truthpart_A_train = os.path.join(root,'part_A_final/train_data','images')part_A_test = os.path.join(root,'part_A_final/test_data','images')part_B_train = os.path.join(root,'part_B_final/train_data','images')part_B_test = os.path.join(root,'part_B_final/test_data','images')path_sets = [part_A_train,part_A_test]img_paths = []for path in path_sets:for img_path in glob.glob(os.path.join(path, '*.jpg')):img_paths.append(img_path)for img_path in img_paths:print(img_path)mat = io.loadmat(img_path.replace('.jpg','.mat').replace('images','ground_truth').replace('IMG_','GT_IMG_'))img= plt.imread(img_path)k = np.zeros((img.shape[0],img.shape[1]))gt = mat["image_info"][0,0][0,0][0]for i in range(0,len(gt)):if int(gt[i][1])<img.shape[0] and int(gt[i][0])<img.shape[1]:k[int(gt[i][1]),int(gt[i][0])]=1k = gaussian_filter_density(k)# plt.subplot(121)# plt.imshow(k)# plt.subplot(122)# plt.imshow(img)# plt.show()with h5py.File(img_path.replace('.jpg','.h5').replace('images','ground_truth'), 'w') as hf:hf['density'] = k# #now see a sample from ShanghaiAplt.imshow(Image.open(img_paths[0]))gt_file = h5py.File(img_paths[0].replace('.jpg','.h5').replace('images','ground_truth'),'r')groundtruth = np.asarray(gt_file['density'])plt.imshow(groundtruth,cmap=CM.jet)#now generate the ShanghaiB's ground truthpath_sets = [part_B_train,part_B_test]img_paths = []for path in path_sets:for img_path in glob.glob(os.path.join(path, '*.jpg')):img_paths.append(img_path)for img_path in img_paths:print(img_path)mat = io.loadmat(img_path.replace('.jpg','.mat').replace('images','ground_truth').replace('IMG_','GT_IMG_'))img= plt.imread(img_path)k = np.zeros((img.shape[0],img.shape[1]))gt = mat["image_info"][0,0][0,0][0]for i in range(0,len(gt)):if int(gt[i][1])<img.shape[0] and int(gt[i][0])<img.shape[1]:k[int(gt[i][1]),int(gt[i][0])]=1k = gaussian_filter(k,15)with h5py.File(img_path.replace('.jpg','.h5').replace('images','ground_truth'), 'w') as hf:hf['density'] = k
参考
https://github.com/leeyeehoo/CSRNet-pytorch/blob/master/make_dataset.ipynb
https://blog.csdn.net/qq_40356092/article/details/108140273